RECOMMEND
Agent
Hi, I am a developer who have some basic knowledge of AI Agent, RAG and Multi-Agent Dialogues. Now I have a small project on hand, which I need to build a AI Agent on the finance industry to give some realtime information to my clients. When I am choosing different AI Agent platforms, I have some difficulties. Right now, I am comparing among Google Vertex AI Agent Builder, Microsoft Azure AI Agents and Salesforce AI Agents. Any suggestions or some free AI Agent Builder recommendations?I am actually cost-sensitive, for example, Google Vertex AI Agent builders have price is $12 per 1,000 queries, and Vertex AI search is $2 per 1,000 queries, which is a little bit above my budget limit.
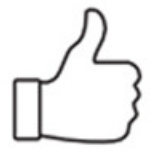
AI Store
大家在使用拼多多(PPD Temu)APP的搜索推荐Search and Recommendation 功能的时候遇到了哪些好的体验和有问题的体验?请麻烦写明复现条件,比如prompt输入文本,上传截图。
大家在使用支付宝(Alipay)APP的搜索推荐Search and Recommendation 功能的时候遇到了哪些好的体验和有问题的体验?请麻烦写明复现条件,比如prompt输入文本,上传截图。
大家在使用淘宝(Taobao)APP的搜索推荐Search and Recommendation 功能的时候遇到了哪些好的体验和有问题的体验?请麻烦写明复现条件,比如prompt输入文本,上传截图。
大家在使用京东(JD)APP的搜索推荐Search and Recommendation 功能的时候遇到了哪些好的体验和有问题的体验?请麻烦写明复现条件,比如prompt输入文本,上传截图。
大家在使用知乎(Zhihu)APP的搜索推荐Search and Recommendation 功能的时候遇到了哪些好的体验和有问题的体验?请麻烦写明复现条件,比如prompt输入文本,上传截图。
大家在使用微信(WeChat)APP的搜索推荐Search and Recommendation 功能的时候遇到了哪些好的体验和有问题的体验?请麻烦写明复现条件,比如prompt输入文本,上传截图。
大家在使用小红书(Xiaohongshu)APP的搜索推荐Search and Recommendation 功能的时候遇到了哪些好的体验和有问题的体验?请麻烦写明复现条件,比如prompt输入文本,上传截图。
大家在使用快手(Kuaishou Kwai)短视频的搜索推荐Search and Recommendation 功能的时候遇到了哪些好的体验和有问题的体验?请麻烦写明复现条件,比如prompt输入文本,上传截图。
OTHER
大家在使用抖音视频的搜索推荐Search and Recommendation 功能的时候遇到了哪些好的体验和有问题的体验?请麻烦写明复现条件,比如prompt输入文本,上传截图。
In this blog, we will give you a brief introduction of most recent progress in On-Device Recommendation (Edge Recommendation) in real-world applications. Mobile AI systems and applications have been more popular due to increasing number of mobile devices and technology developments in deep learning based methods, e.g. model compression, distillation and so on. In recent years, on-device recommendations have enpowered many Mobile Apps to better respond to users' most real-time behaviors on mobile deivces, including clicks, scroll-donwns, likes, and many others. We will introduce three applications, including EdgeRec in Taobao, searchbar background words reranking in Alipay, search result reranking in Meituan-Dianping, short-video recommendation in KuaiShou, TfLite Implementation of Tensorflow, etc.
Ranking is a crucial part of modern commercial recommendation and advertisement system. It aims to solve the problem of accurate click-through rate(CTR) prediction. In this article, we will provides some of most popular ranking equations of commercial recommendation or ads system.
This blog summarizes the latest research development of Retrieval papers published in KDD2023 conferences. This year there are total 8 papers related to Retrieval in KDD2023. Most of the authors' affiliations are top research institutes (Google Research, DeepMind, Meta FAIR) and universities (Stanford, Berkeley, MIT, CMU and others).
This blog summarizes the latest research development of Recommendation papers published in KDD2023 conferences. This year there are total 44 papers related to Recommendation in KDD2023. Most of the authors' affiliations are top research institutes (Google Research, DeepMind, Meta FAIR) and universities (Stanford, Berkeley, MIT, CMU and others).
Cross-Domain Recommendation in Commercial Recommendation System With application of MMD and Wasserstein distance
In this post, we will talk about some real-world applications of deep candidates generation (vector-retrieval) models in the matching stage of recommendation scenario. Commercial recommendation system will recommend tens of millions of items to each user. And the recommendation process usually consists of two stages: The first stage is the candidate generation(matching) stage, a few hundred candidates are selected from the pool of all candidate items. The second stage is the ranking stage in which hundreds of items are ranked and sorted by the ranking score. Then the top rated items are displayed to users.